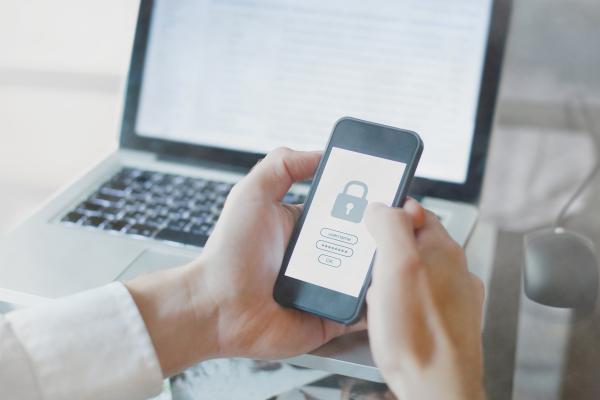
Back in February 2023, Google’s chatbot Bard incorrectly suggested that the James Webb Space Telescope was first to take pictures of a planet outside the Earth’s solar system. This mistake caused Alphabet’s share prices to drop, along with Bard’s credibility.
These kinds of errors point to the importance of high-quality data in ensuring that Gen AI models, such as Bard or ChatGPT, provide reliable and accurate outputs.
High-quality training data is essential for making sure that AI and large language models (LLM), like ChatGPT, provide reliable and accurate outputs. Poor data quality can lead to several issues with machine learning, including hallucinations – generating information that is not based on reality – misinformation, lack of context, and unintended bias.
WHAT ARE AI HALLUCINATIONS?
AI and LLM hallucinations take place when an AI model generates content that appears plausible but is actually incorrect or fabricated. These hallucinations occur when the model generates information that it has not learned from the training data but, rather, generates through patterns it has detected – and these may not always be accurate. AI hallucinations can include the aforementioned historical inaccuracy, generating non-existent references or citations, or inventing erroneous details in a query response.
WHAT CAUSES AI HALLUCINATIONS?
AI hallucinations can be caused by a range of issues. These include errors, inconsistencies, or outdated information in the training data, inherent limitations in the AI’s architecture or algorithms, and missing data validations. Inadequate data governance (such as poor-quality policies and practices for managing data integrity) exacerbates this problem as there are no suitable structures to control data input and ensure data quality at the outset.
In the real world, AI hallucinations can have serious repercussions.
AI in healthcare, for example, has the potential to improve care and reduce professional burnout. Integrating AI-based chatbots into healthcare advice can increase productivity and reduce costs dramatically. But AI hallucinations can result in misdiagnoses and incorrect medical advice. Research has shown that without careful monitoring by human healthcare professionals, AI algorithms can perpetuate existing biases, leading to unequal access to care, misdiagnoses, and inadequate treatment recommendations.
AI hallucinations can contribute to the spread of misinformation, and this poses a real threat to democracy. Additionally, AI hallucinations can result in inaccurate investment or financial guidance, having implications for company and stock market stability. There’s also some evidence that biased training data can help perpetuate gender or racial biases, as well as being able to produce harmful or offensive material.
LESSONS LEARNED - HOW TO MITIGATE AI HALLUCINATIONS
Ensuring the accuracy, completeness, consistency, relevance, validity, and timeliness of training data is key. This can be achieved through diverse data sources (incorporating a wide range of data to reduce biases and improve generalization), regular updates of the training data to reflect the latest information, and ongoing testing and evaluation.
Continuous monitoring and testing of AI models can help identify and rectify hallucinations. Techniques include benchmarking, that is comparing AI outputs against a set of standards or gold standards, and stress testing, that is evaluating the model’s performance under various scenarios to identify weaknesses.
The human touch
Involving human experts to oversee and validate AI outputs can significantly reduce the risk of hallucinations. This includes regularly reviewing AI-generated content for accuracy and relevance and incorporating user feedback to refine and improve models. Engaging users in providing feedback on AI outputs can also help identify errors and improve the model. This involves allowing users to report inaccuracies or issues with AI responses and using iterative feedback to continuously refine and enhance the model.
DATA GOVERNANCE
Implementing robust data governance practices ensures that data management policies are adhered to, enhancing data quality. Key components of good data governance include processes such as data validation – establishing checks to verify data before it is used for training – and data stewardship – assigning responsibility for maintaining data integrity and quality.
Mitigating AI hallucinations requires a comprehensive approach focusing on data quality, continuous evaluation, and human oversight. By ensuring training data is accurate and diverse, we can help reduce bias and enhance model reliability. Combining this with robust data governance, including strict data validation and stewardship, will help create a comprehensive framework for minimizing hallucinations and ensuring more trustworthy AI.
SPS GPT
Integrating a conversational interface that accesses a pre-established internal directory of information, providing an intuitive user-friendly platform to interact with through queries and answers. The model can be exponentially trained to behave more accurately day by day based on every client’s needs.
As part of our goal of leading the way, we apply the artificial intelligence and machine learning benefits to our Hybrid Workforce, Office Logistics and Business Support solutions, including custom GPT interfaces capable of being trained on any specific data model.
RELATED ARTICLES
The importance of good data management
The successful use of GenAI solutions really hinges on a robust data management strategy to ensure accuracy, security and efficiency of the data used, as the possibilities are endless.
Prompt engineering
Publicly available generative AI applications are now creating output that is virtually nearly indistinguishable from human efforts.
Revolutionize workplaces and with Generative AI
Generative AI is redefining how we communicate, create, learn, and interact with technology. This disruptive innovation will massively change our lives, it will revolutionize workplaces and trigger new business models.